Document Intelligence Centre of Excellence
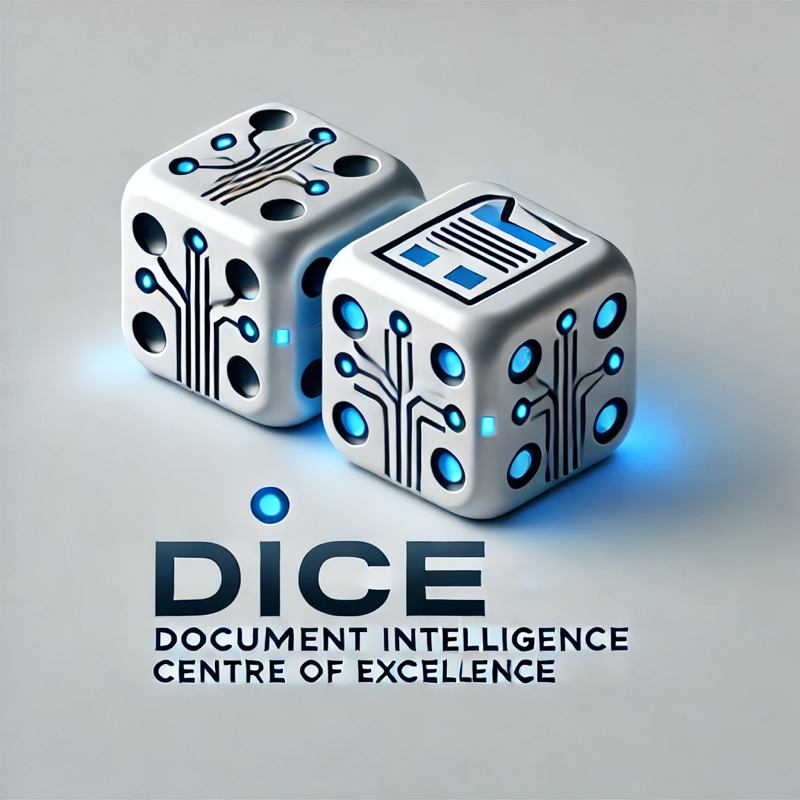
Patr. Gregoriou E & Neapoleos Str 27
15341 Agia Paraskevi
Athens, Greece
DICE is a Document Intelligence Centre of Excellence. Its mission is to develop novel AI methods and become a world class reference centre in AI for business documents.
Its vision is to connect researchers, scientists and AI professionals with business experts from a wide range of industrial sectors, aiming at using emerging technologies to accelerate innovation.
Research synopsis: Our principal research interests lie in the development of machine learning and statistical methodologies for solving problems involving un-/semi-/fully-structured financial data.
We are part of the Institute of Informatics and Telecommunications (IIT) at the National Center for Scientific Research - NCSR “Demokritos”.
Check out our publications and our projects outreach to learn more abour our work and feel free to reach out, if you are interested!
News
Jun 20, 2025 | DICE will be presenting in the 38th IEEE International Symposium on Computer-Based Medical Systems (CBMS), its latest work on Predicting Multi-Class Drug-Drug Interactions Using a Disease-Specific Knowledge Graph💊 as a full oral! Stay tuned for the full paper! |
---|---|
Apr 24, 2025 | DICE placed 1st in the Field of Research Classification shared task, hosted by the Natural Scientific Language Processing and Research Knowledge Graphs (NSLP 2025) workshop @ESWC2025 🏆🎉🎉! Stay tuned for the corresponding paper, utiling LLMs for zero-shot classification! |
Apr 15, 2025 | DICE participated in the Pharos AI Factory kick-off meeting, one of Europe’s first 13 AI Factories🏭! See more details here!🎉 |
Mar 20, 2025 | Our latest survey: Machine Learning for Identifying Risk in Financial Statements: A Survey has been just accepted in ACM Computing Surveys🎉! |